AI-based Price Optimization for DIY Retailer
Learn how a DIY retailer maximized revenue without traffic losses in the short term and saved profit during the low season.
Key results after the Proof of Concept stage
12.3%
Revenue uplift
8.45%
Gross profit uplift
6.31%
Increase in sales items
Client
Trading house Vimos is a Russian retail chain specializing in DIY goods and construction materials. The company operates in Russia's North-West regional market. So far, Vimos has 43 trading centers offering more than 25 000 SKUs. The retailer's annual turnover goes beyond $160 million.
Context. In 2020, Vimos launched a price optimization project in cooperation with KORUS Consulting – a group of IT companies advising retailers on pricing strategies, profit and business management since 2000. KORUS team proposed Vimos to build a solution based on automated pricing powered with artificial intelligence. They needed a solution that could deliver quick and measurable results being flexible in terms of integration and customization. Competera met all the requirements and proposed to run a pilot on a limited number of stores to prove its effectiveness.
Сhallenges
-
Seasonal spikes in demand.
-
Integration with existing legacy ERP system.
-
The solution must be simple and easy to understand for each member of the category management team.
Goals
-
Maximize profit and revenue without traffic losses in the short and long term.
-
Save profit during the low season.
-
Provide a comprehensive solution based on predictive principles for managing pricing tactics and strategy.
-
Accelerate all repricing processes with a centralized price management tool.
Solution
Integration
Seamless integration of pricing solutions like Competera directly depends on the quality of the retailer's data. The data set must be complete, consistent, and regularly updated. To meet these criteria, the project team started with structuring and cleaning up the historical data set provided by Vimos.
Since the client uses an in-house ERP, this stage also implied integrating the pricing platform into the existing client's system. The complex data exchange process was set up (including the daily automatic validation process).
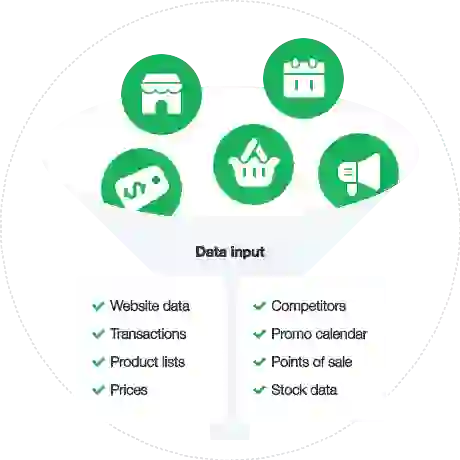
Onboarding
After the integration, key persons involved in repricing, i.e., 7 product managers, were onboarded and introduced to the platform functionality. The process included understanding the system's capabilities, adjusting, and learning how to correctly interpret the proposed pricing recommendations.
At the same time, the Competera team began training neural networks on client data.
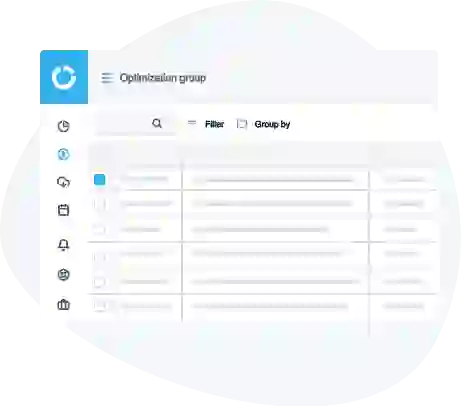
Pilot
To measure the effectiveness of Competera, the POC stage took place on a formed test group with following details:
Test group:
5 hypermarkets or 10% of the retailer's geography
Control group:
5 hypermarkets with standard repricing process
Repricing was performed for the entire assortment at each point of sale (25,000 SKUs)
KVI and promo products were excluded for repricing by Competera
Group selection methodology: the selection included stores that, on the one hand, were representative for the Vimos chain and, on the other hand, had the most comparable structure of demand (by average check, revenue, number of SKUs) and the dynamics of metrics changes. The pilot's duration was one quarter.
Pricing recommendations architecture
The artificial intelligence-based system calculated the elasticity of demand for each product based on internal (historical) and external data, analyzed it, and gave price recommendations for products at each point of sale. You can see an example of the optimization curve for one of the categories on the left.
It is important to note that price recommendations shouldn't be considered as an optimal price for each SKU individually. Instead, each price optimization result is a set of optimal prices for all SKUs in the optimization group. Usually, one group represents one product category. This approach allows you to predict and achieve the target result more effectively. The main goal of Vimos was the maximization of profit and revenue.
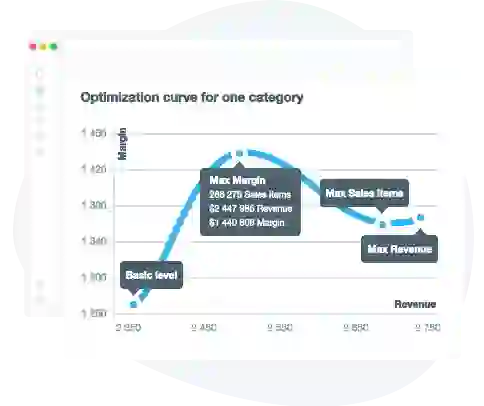
Pricing campaigns' management
A comprehensive price management approach combined with platform settings' flexibility allowed the client to overcome seasonal sales spikes (summer holidays are traditionally marked by a sales increase in the DIY industry). Due to these factors, Vimos smoothly entered a pandemic and the end of the business season, usually characterized by a drop in sales.
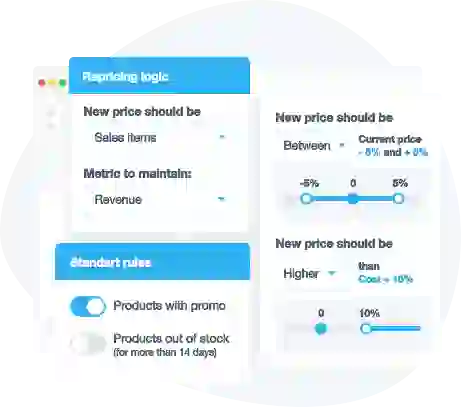
Results
The proof of Concept stage took one quarter. The test group exceeded the control group by all parameters.
Revenue increased by 12.3%
Gross profit boosted by 8.45%
Sales items increased by 6.31%
1% of traffic
growth
Repricing takes 2 hours once a week
The indicators above represent the difference in the dynamics of sales indicators between the test and control groups. The dynamics mark the change in the indicators in the test period compared to the control period. The test period is 17 weeks, during which the test group was repriced using the Competera solution while the control group was repriced in a usual way. The control period is a pre-test period during which repricing was performed in the same way in both test and control groups.
Competera and KORUS Consulting reached all targets
After the pilot launch, Vimos decided to scale Competera on all 43 stores and update its pricing methodology and approaches. Together with the partners, they develop a complex pricing system considering price zones, KVIs, optimization strategies, etc. Here are the next stages of the project.
Revision
and configuration of optimization groups
Shaping
price zones and KVI list using ML algorithms
Setting up
the competitors’ monitoring
Development
of dashboards for advanced analysis
Setting up
Competera for the new products' pricing
Competera Pricing Platform helps retailers to craft optimal offers
Want to give feedback or ask a question? Email us at [email protected]